A cyclical service delivery cascade in the context of HIV
How does changing one tiny bit of a service delivery cascade change the way we think about it? Plus the cost-effectiveness of gene therapies, consumer trust in storing health data, and nudging old people to write advance directives for EOL care.
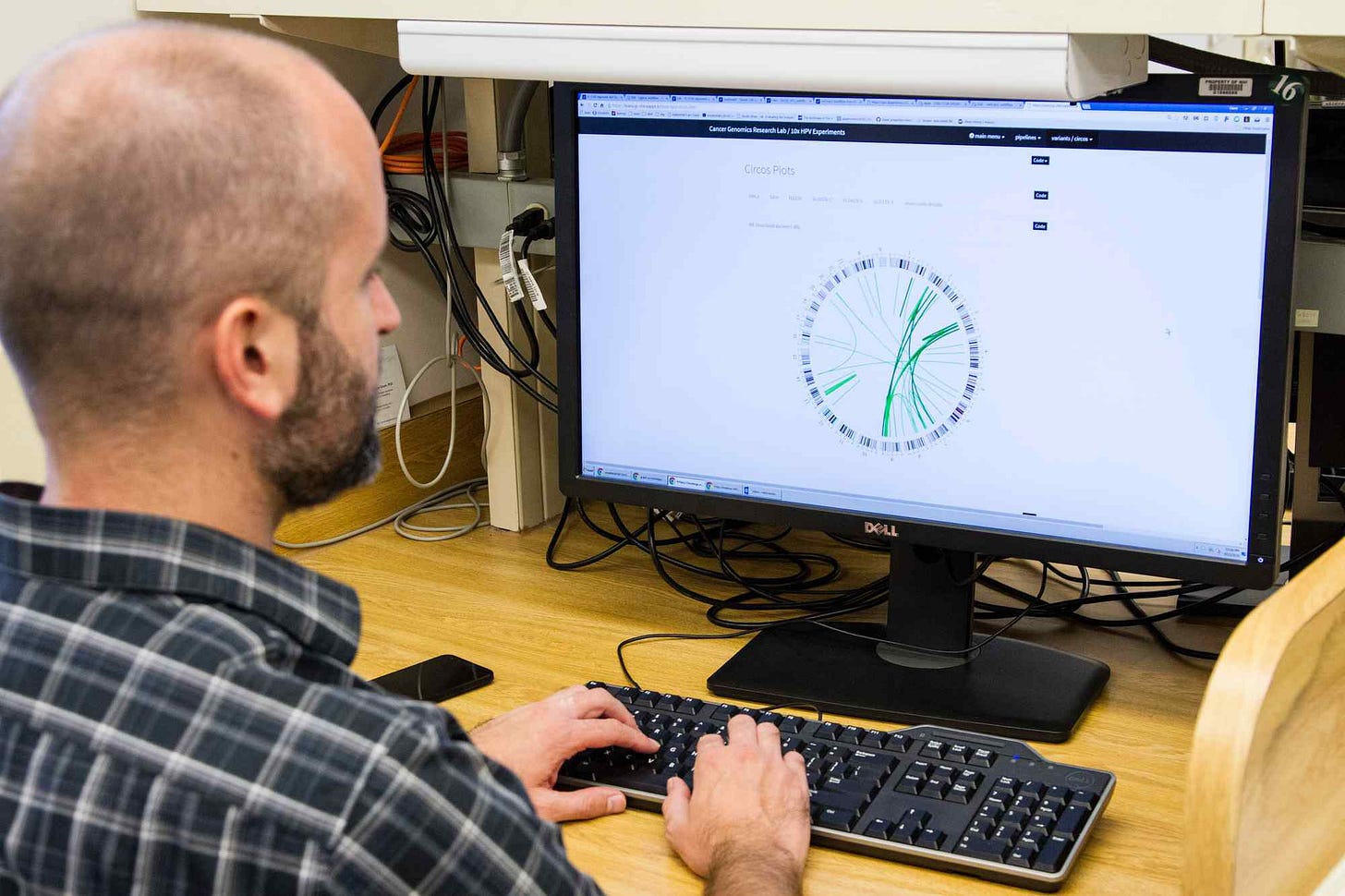
The global HIV community, in 2014, adopted a target principle called 90-90-90: the diagnosis of 90% of people living with HIV (PLHIV), antiretroviral therapy (ART) for 90% of those diagnosed HIV–positive, and viral suppression in 90% of those receiving ART by 2020. These goals were revised up to 95-95-95 by 2030. However, we remain quite a distance from where we ought to be at this time. We are currently at 81-82-88, which gives us an overall viral suppression rate of only 59%, a long way from where we ought to be.
Why is this so? What is the point at which people stop being part of the system? Ehrenkranz et. al. (2021) point out that most people who get diagnosed with HIV do not tend to drop out before the start of ART because of a push to begin ART on the same day as diagnosis. Thus it becomes important to understand where people actually decide to disengage and what the best method for getting these people back into the system would be.
Typically, most models which aim to show the system for dealing with HIV are linear with a person entering through one end and exiting at the other due to death or loss of follow up. However, that is not how these systems work: people can leave at any point. People tend to enter and exit the system at various points. As the authors point out:
Routinely collected medical records in most settings do not adequately document this phenomenon. In one notable exception, South Africa’s Western Cape Province undertook a pilot that used unique patient identifiers and digitized routinely collected point-of-care HIV test results to assess testing and restarts. Within their intervention site, 51% of people who tested HIV–positive had previously been diagnosed, and 71% of these had previously started ART. In other words, more than one-third of HIV testers had previously been on ART. This information was used to highlight the need to redirect resources from expansion of HIV testing to improved focus on continuity of care.
Any model which aims to properly model an HIV intervention ought to take this into account.
In order to do that, the authors propose the creation of a cyclical cascade model.
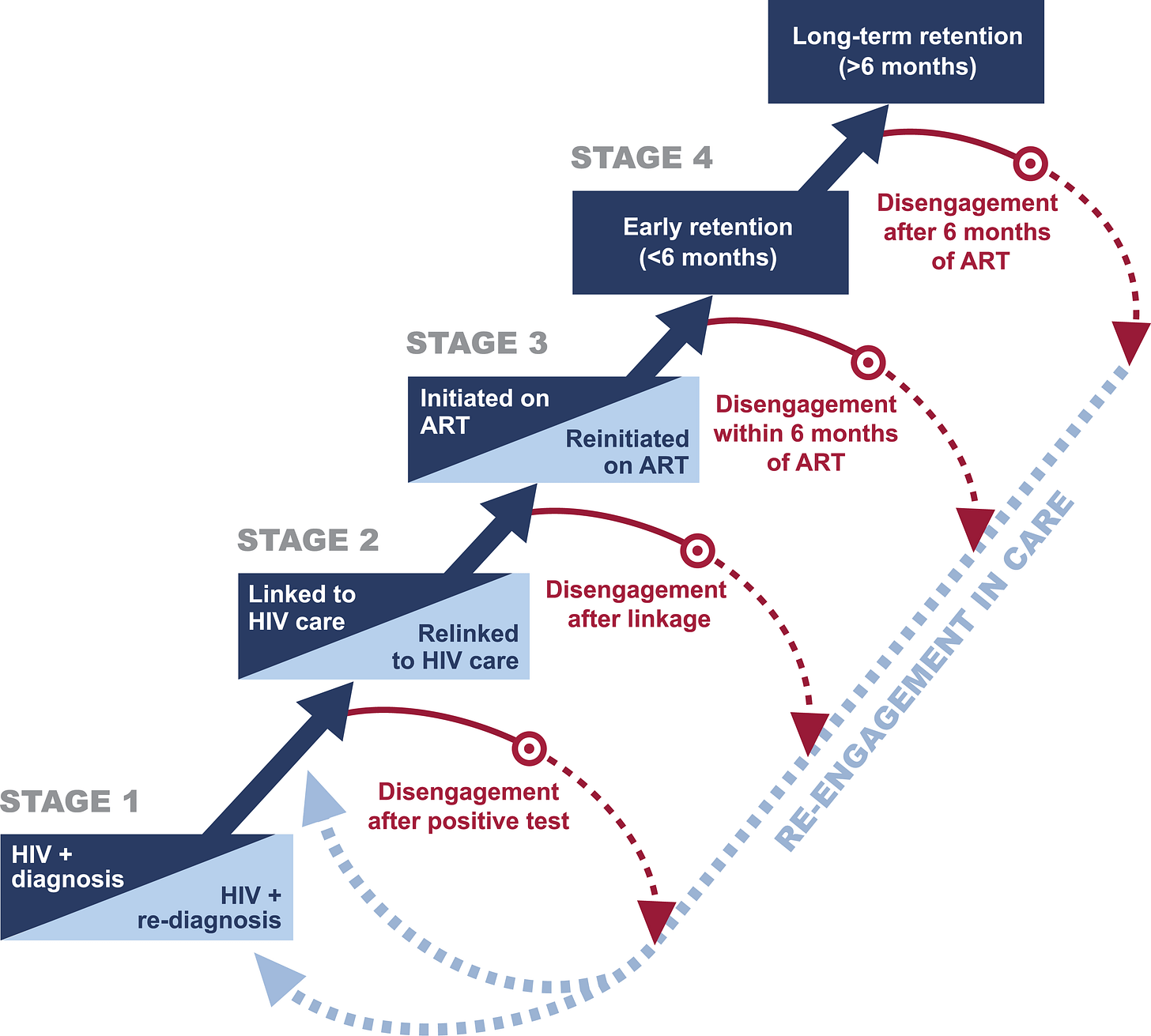
This model has four stages with the addition of a disengagement step as well. The primary advancement in using such a model, simple as it may sound, is that one can now model disengagement at any step. This can make it easier (institutionally) to understand the stages at which patients tend to have the greatest tendency to drop out as well as the frequency of a patient's drop-out at each stage. Further it also allows one to understand the effect of each stage on on return (when it happens) and the implications of loss or reentry at each stage on future stages. The authors do note:
Clinical outcomes, such as viral load suppression, are not explicitly included in the cascade as long-term retention in care, the fourth stage in our cascade, is highly correlated with suppressed viral load, and our intention is to better understand PLHIV behavior as it relates to patterns of engagement, not the biological results of treatment.
Policy Implications
This cascade may be used to distribute resources more efficiently. Different kinds of interventions may need to be targeted at people who disengage at different stages, something this model explicitly enables. It will make it possible to figure out populations most likely to disengage and help their retention in care, figure out people who have disengaged and work towards re-engaging them with the process, and most importantly, identifying sub-populations which are susceptible to dropping out at any given stage. Targeting entire populations without tailoring interventions towards those who explicitly need them is a waste of resources because majority of people who are diagnosed and start ART do not leave it.
Of course, putting this cascade in action will require specialised data from clinical interventions, surveys, and population surveillance. This data will differ from country-to-country and can be used to tailor the cascade according to the needs of the area and the population. Specifically, the integration of electronic medical records can be used to track people who fall through the system (for example people who were previously diagnosed but decided to stop taking ART need not be diagnosed again).
This model may evolve differently within countries. For example, if transportation to the clinic for conducting ART is a major bottleneck that may be added to the model. If it has been found that reintegrating people who were previously in the system is best done by diagnosing them again it would be best if that relationship is added to the cascade. These models will thus change from area to area, country to country and will, after a while, not be applicable to other places without major adaptations. However, they will be extremely valuable locally and have the potential to increasingly streamline existing systems and processes.
Cost-effectiveness of gene therapy for Sickle Cell Disease
Sickle Cell Disease, often confused with Sickle Cell Anaemia, is a group of genetic disorders associated with lifelong complications, a reduced life span, and increased usage of healthcare systems. The clinical manifestation is characterised by damaged and abnormally shaped red blood cells which result in their rupture, the blockage and obstruction of small blood vessels, and other complications resulting from these issues. Severe pain episodes tend to be a common result of sickle cell disease.
Currently, treatment varies according to the age group but typically includes both preventive and palliative care. The most common forms of treatment include vaccination, hydroxyurea, blood transfusion, penicillin, and opioids. Hydroxyurea has been found to be tolerated fairly well in patients of all ages: however, it has many side effects and patients and providers both often skip taking it due to concerns about long-term risks.
On the other hand, there are a few other therapies available. Haematopoietic Stem Cell Transplant is a potentially curative treatment but is typically reserved for those with extremely severe forms of the disease. It's availability tends to be scarce and it remains associated with many ifs and buts, like the possibility of transplant rejection, long-term issues, and various long-term adverse outcomes.
Salcedo, Bulovic, and Young (2021) focus on a new gene therapy targeted at these patients and check whether it falls within current thresholds of cost-effectiveness or not. The treatment involves removing haematopoietic stem cells from a patient, modifying the gene responsible for sickle cell disease to produce an anti-sickling haemoglobin B, and then reintroducing these cells into the patient. Initial trials of this therapy showed substantial improvements within 3 months of it being introduced.
The authors utilised a Markov Model to estimate the incremental cost-effectiveness ratio (ICER) of the treatment. Based on their analysis, they showed that an average patient with sickle cell disease stood to gain 8.5 quality-adjusted life years (QALYs) and 3.7 life years (LYs) over their lifetimes at an incremental cost of $1,196,917 over a lifetime. This assumes a $2.1 million cost for the treatment. Accounting for gender, it was found that this translated to around $146,511 per QALY and $135,574 per QALY for females and males respectively. However, this presumed that a single administration of the therapy would work for all patients. In case 50% of the patients were to relapse in 20 years, the ICER of this treatment goes up to $410,607 per QALY. If the relapse rate were to be 50% in 10 years instead, this increases to $740,058 per QALY.
It was also found that if one were willing to pay around $250,000/QALY for this treatment, then the chance of this treatment not being cost-effective decreases dramatically. The authors further found out that the treatment would be cost-effective 32.7%, 66.0%, and 92.6% of the time at values of $100,000, $150,000, and $200,000 per QALY, respectively.
There are a few limitations of this study:
The authors assume that the therapy is provided at birth, which might not happen for a variety of reasons even in developed countries
Their most optimistic assumption is that the therapy is 100% effective. However, until we have more data, this remains an extraordinarily optimistic scenario
The authors do not consider a societal perspective here and look only at direct medical and healthcare costs. The costs borne by society (caregivers, workplace, etc.) are not considered
This study was conducted looking at a single specific treatment called Zolgensma
The price of this therapy may be determined by market prices. This price differential might be enough to tip this therapy into being cost-ineffective
The acceptance of gene therapy amongst Americans is also unclear. It would be wise to address mistrust in the health system and suspicion towards genetic therapies in general while introducing this treatment option
Using Behavioural Economics to promote advance directives for EOL care
Over the course of a lifetime, individuals make many different choices about their healthcare. One of the most important choices they have to make nearing the end of their lives is that about provision of care towards the end. While an extremely important task, surveys of nearly 800,000 American adults in aggregate showed that only around 30% of them had taken part in this activity. This proportion has changed very little across the first half of the 2010s and numerous legislative and research initiatives have done little to change this.
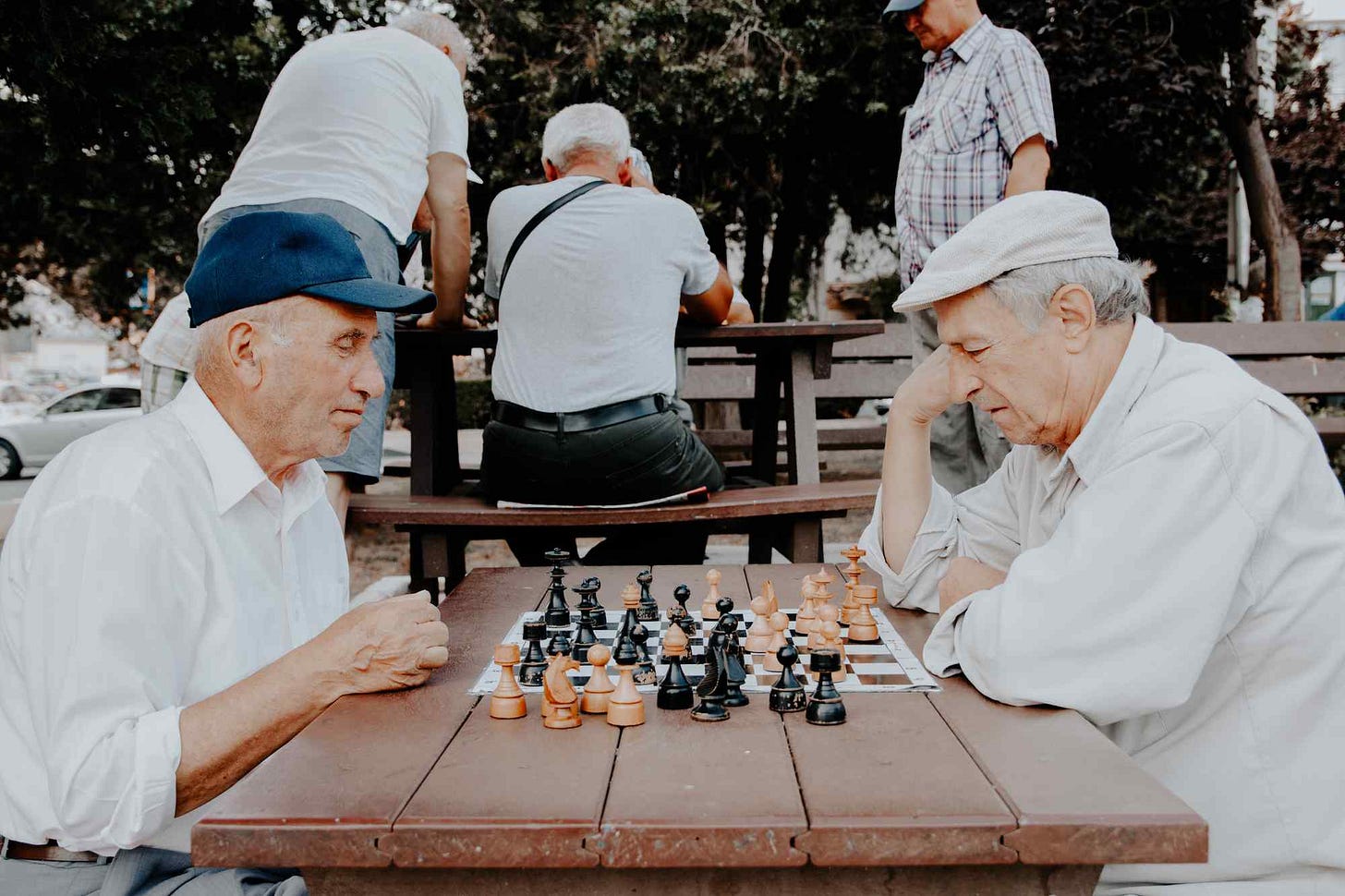
Spivey, Brown and Courtney (2021) apply the logic of Behavioural Economics to see what kind of messaging makes the idea of advance directives (ADs) stick in the heads of older people. Prior research has found that the most important reason to go for ADs at EOL is to reduce the burden on family members. This trumps financial autonomy as well. It has also been found that an individual with defined ADs is likely to incur lower costs during their EOL times and have a lower chance of dying in a hospital rather than at home. The primary reason why people reported as not having made an AD is because they believed that their loved ones already knew their wishes.
In order to check what may motivate someone to create ADs, the authors look at the idea of framing.
Framing, a behavioural economics technique, posits that the way in which information is delivered matters to how individuals make choices. For example, the likelihood of an individual choosing to have a surgical procedure will differ depending on whether they are told the procedure has a 5% mortality rate (negative or loss-framed) or that it has a 95% survival rate (positive or gain-framed). Prior studies of framing related to health have shown that positive frames are more effective for prevention, while negative frames are more persuasive for detection.
In order to test the effects of framing on people, the authors recruited older people who had not yet defined their ADs. After an initial survey, they were shown an informational video about AD. A survey was conducted after this, and then they were divided into groups and shown one of three videos:
There is a 1 min and 28 s negatively framed video that features a brother, sister and sister’s husband in a hospital waiting room trying to make an end-of-life care decision for their father who is incapacitated and who did not have an advance directive. This family is in grief and worries over the decisions they should make given they don’t know their father’s wishes (we will refer to this video as negative framed video)
Another video is a 1 min and 7 s positively framed video that features the same family in the previous video. This time their father had an advance directive, so while in grief, they are relieved their father’s wishes are carried out (we will refer to this video as the positive framed video)
The final 1 min and 37 s video uses a social norm to frame the decision to make an advance directive. This video features two friends discussing a third friend’s experience when her husband was incapacitated but had no advance directive. The friends conclude an advance directive is a good thing and after doing research have decided to get one, too
The participants were asked survey questions after watching the video. The participants were then shown the other two videos (in different orders for different groups) and asked survey questions after each one.
The authors find, not surprisingly, that negative framing of this message is correlated with efforts to get more information about ADs. For people who had not sought information about ADs prior to the study, positive framing was seen to have a small positive impact on the approximate change in creating ADs. The authors seem to conclude that negatively framed videos might be better at getting people to get more information about ADs, and positive messaging may be better at getting them to actually create ADs. Social norm messaging was also seen to be positively correlated with the creation of ADs.
The authors also found that positive messaging around ADs was seen to be more convincing the older a participant was, while negative messaging was more convincing to younger participants. On the other hand, people with a chronic disease were best targeted using social norm messaging.
Policy Implications
It is important for messaging to be tailored to the audience: this is true for COVID-19 vaccination messaging as well as for creating advance directives for EOL care. It would be a good idea to incorporate training in behavioural economics for both doctors and nurses in order to get them to understand how to convince or nudge people towards any specific health policy. This information also ought to be considered when creating government campaigns about specific diseases.
Customer trust in public and private organisations in storing COVID-19 data
A huge problem in combating the COVID-19 pandemic today is consumer trust. Consumer distrust manifests itself in a lot of ways, be it vaccine hesitancy, refusal to trust authority figures on the actual reach and impact of the pandemic, or a refusal to share data about one's own health.
Grande et. al. (2021) take a look at the last issue. They administered a survey to a group of US respondents to see their levels of trust in various organisations when it came to keeping track of COVID-19 data. The respondents were asked to rate nine types of organisations:
University Researchers
State Health Department
Local health department run by a your county/city
Health Agency at the federal government
Health Insurance Company
A company that makes digital thermometers
Apple
Google
Facebook
It was found that respondents actually had the strongest confidence in university researchers followed by State or local health departments. Corporations were uniformly ranked as the least trustworthy. The authors also state:
In multivariable models estimating the proportion of consumers that were at least somewhat confident in public health agencies, there were large differences by political ideology of the respondent—71% of self-identified liberals expressed confidence in state health departments to protect digital health information compared to 57% of moderates (p<0.001) and 45% of conservatives (p<0.001). These differences were similar for local public health departments and federal health agencies. We did not observe greater confidence among those with a family history of COVID-19 or those living in a higher incidence county. We did not find significant differences between Black vs. White respondents. Hispanic respondents reported higher confidence than non-Hispanic respondents (61% vs. 56%, p=0.04). Differences by income, geography, and age were small and generally not statistically significant.
The authors also report that the only variable they find really affecting these responses are the political beliefs of the respondents: no other variable seemed to make a difference.
Policy Implications
While the polarisation of society and its effect on vaccine skepticism and mask-wearing is well-known, it seems these effects extend to cover the stewardship of data as well. The agencies responsible for collecting data and analysing it would do well to alter their messaging and communicate properly with all sections of society. Prior research has shown that consumers are very particular about data protections and privacy: it might be a good idea to be very clear in the communication of the same to the general public. In addition, the usage of services by private companies may have the effect of pushing people away from sharing their data: it would be a good idea for governments to either build this data collection and analysis capability in-house, or bring it within the ambit of the HIPAA or related laws. That would go a long way towards reassuring people about the safety of their data.
The effect of Customer Directed Care (CDC) on Mental Health in Australia
Australia has been promoting the use of a model called Customer Directed Care. In the words of Ausmed:
Consumer-directed care (or ‘CDC’), refers to a ‘self-directed’ healthcare model in which the client is afforded the right to full autonomy in all decision-making related to that care. Historically, these care decisions would be made by the care team, however CDC empowers the care recipient by aiming to improve their health literacy, so that they can play an active role in their care pathway (Ansara 2014). All home care packages in Australia are currently mandated to be based on a consumer-directed care model, fulfilling Standard 1 of the Aged Care Quality Standards: Consumer Dignity and Choice (My Aged Care 2015).
Specifically, this model provides greater choice to the customer: while it remains the care team's responsibility to give recommendations to the customer and enable them to make choices about their own healthcare, the final responsibility lies with the customer himself or herself. The care recipient has the right to delegate as much or as little of the decision-making to care providers as they wish.
Specifically, Australia follows this model in all home care packages (HCPs) as well. Home Care Packages are meant for older people who wish to receive healthcare without being shifted out of their homes into an external care home. Unfortunately, the mental health effects of the model in this context have not been studied quantitatively before. Tran and Gannon (2021) set out to fill that hole in public policy literature. They analysed data on 1780 individuals aged 65 years and older across 11 iterations of the Household, Income and Labour Dynamics in Australia (HILDA) Survey. To measure mental health, they used the MHI-5 scale.
The MHI-5 scale is constructed from the following items in which respondents answers to how often in the past 4 weeks they have (1) been nervous, (2) felt so down in the dumps that nothing could cheer them up, (3) felt calm and peaceful, (4) felt down, and (5) been happy. Answers to each item were coded on 1 to 6 scales where 1 represents “all the time” and 6 represents “none of the time”. All scores across each item, are summed up, then subtracted by 5 and divided by 25 to derive a 0-to-100 mental health scale.
Specifically, they find that older adults tend to have significantly worse mental health outcomes after the introduction of the CDC model (which was introduced in 2013). To make sure that this actually corresponded with the uptake of Home Care Packages, they looked at mental health scores across regions and found that regions with higher uptake of HCPs tended to have worse mental health outcomes.
In addition, the authors find that this tends to vary across educational levels as well, which they posit might be because education corresponds to financial literacy, which may cause less educated people to take on greater stress when it comes to managing their care. Similarly, access to care might be harder in non-urban areas causing more stress to rural residents. And finally, the gender disparity seen in stress levels (men are less likely to report good mental health than women) was posited by the authors to be because men tend to live in more rural areas than women in their specific sample.