Generative AI in healthcare
Generative AI has a bright future in medicine, with GANs emerging as a powerful tool in harnessing computation to solve fundamental issues in effective service delivery.
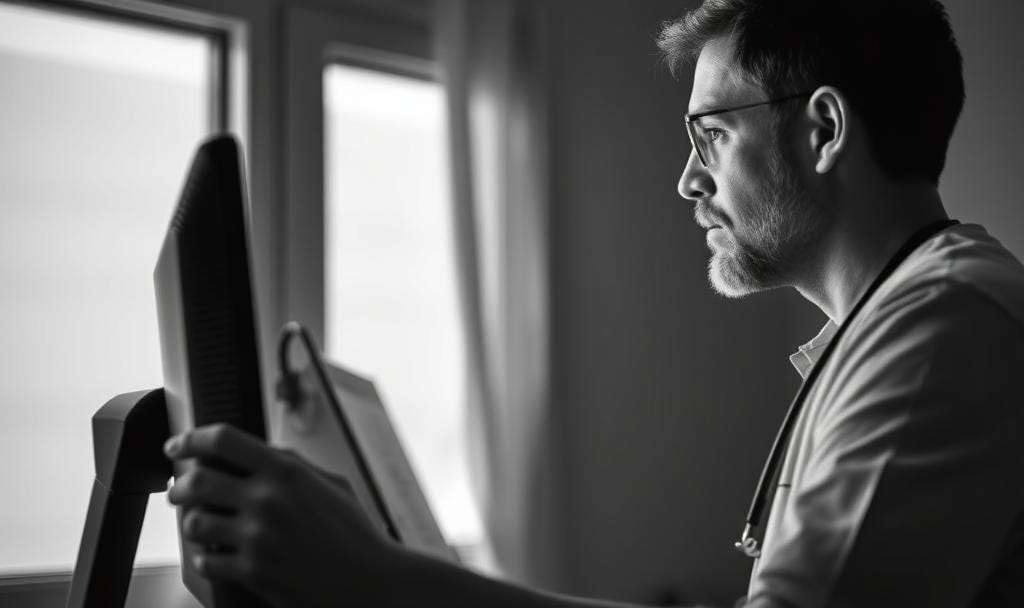
ChatGPT took the world by storm when it came out in 2022. Millions of people thought it was a quantum leap in information processing, generation, and ideation. Hundreds of thousands signed up for a paid ChatGPT account and had a virtual assistant on their fingertips. It was rightly thought of as a Sputnik moment in AI, when the first sparks of consciousness began to slowly light up behind the eyes of formerly inanimate machines.
However, biology and healthcare were a little early to the party. Google released AlphaFold 2 in 2020, which placed first in the Critical Assessment of Structure Prediction (CASP14) that year. The results were seen as ground-breaking and astonishing at the time, even though researchers noted that the accuracy was slightly inaccurate for around a third its predictions. It could also not explain the reasoning behind its structural predictions, which was seen as a major shortcoming.
Fortunately, that was no barrier to its adoption. The AlphaFold paper has been cited more than 32,000 times since it was published in 2021. The medical and biological sciences, while (rightly) careful about accuracy and validity, put great value on utility. This attitude towards AI bodes well for the adoption of new, novel methods of increasing efficiency in both clinical and non-clinical roles, a significant part of which is generative AI.
Generative Adversarial Networks
Generative adversarial networks (GANs) represent a powerful method towards creating new types of generative AI. A GAN comprises of a pair of components which work in tandem to create realistic data and evaluate its authenticity. The two components are a generator network and a discriminator network.
The generator works to create new data samples. It starts off with random noise and trains to create a result which resembles a specific target. In a way, the generator is trying to create a masterpiece by throwing paint at a wall. At first, the generator is very bad at throwing the paint, but with enough practice, a generator can become very good at getting close to the kind of target which we wish to look at.
A discriminator network is the arch-nemesis of the generator. It trains to distinguish between real data and data synthesised by the generator. The discriminator's job is to check the results of the generator and provide feedback, helping it improve over time. Continuing the analogy from before, the discriminator trains to be an art critic on the generator's canvas.
The training process of the GANs starts off with the generator struggling to produce anything realistic and the discriminator being very poor at discriminating. However, repeated iterations lead to the generator improving based on feedback from the discriminator, and the discriminator becoming a better critic of the generator's work. As this back and forth goes on, the networks reach a stage where it becomes difficult for the discriminator to distinguish between real data and data created by the generator. This is called a Nash equilibrium. The generator has learned to generate data which closely matches the target data, and the discriminator is very good at judging between real and fake data.
This process can be difficult: there exists a right balance between generator and discriminator which needs to be hit for the process to make sense of things. The hunt for techniques to improve and streamline this process is a particularly hot topic right now.
Applications of Generative AI in healthcare
This process may seem unimportant for medical technology. After all, what need does the medical field have for generating data? Doesn't medicine have a surplus of data which it cannot handle and/or interpret? Well, yes, and no. While it is true that certain widespread diseases do have more data available than we know what to do with, there are specific diseases called rare diseases where data is scant. GANs have the potential to generate realistic data simulating various rare conditions which can then be used to train diagnostic AI as well as doctors, allowing rarer conditions to more effectively be targeted by practitioners. Similarly, it may be safer to use GANs to simulate virtual patient populations to predict treatment outcomes across diverse social and genetic populations, allowing for a more probabilistic interpretation of the effects of new treatment plans. This is especially important for rare diseases, where insufficient real-world data exists for us to have any idea of the probabilities of various treatment modalities.
Generative AI can also be used to be used to prepare tailored personalised medicine plans. It has shown significant potential in building targeted cognitive behavioural therapy regimens. TP-GAN (treatment planning with GAN) is a framework utilising GANs for automating prostate brachytherapy planning. Generative AI is also used in also assist in diagnosing dental health conditions such as caries and periodontal diseases through image analysis and data interpretation, significantly reducing the cognitive load on dentists and enabling faster onboarding of new staff.
Another way of using generative AI is to use GANs and other technologies to generate synthetic medical data which safely replaces patient information while being able to satisfy laws such as the HIPAA or GDPR. Synthetic data generated this way can be used for training and tool validation. Studies have shown that augmenting real datasets with synthetically created radiographic images using latent diffusion models leads to improved classification performance by diagnostic AI algorithms.